When it comes to data visualization and analysis, Orange has long been a trusted companion for professionals and enthusiasts alike. Its user-friendly interface and powerful features have made it a staple tool in the data science community. However, just like any other software, Orange has its own set of features and limitations. In this article, we’ll delve into the world of Orange alternatives, exploring their features, limitations, and what sets them apart. So, why the need for an Orange alternative? Let’s find out.
The Power of Orange
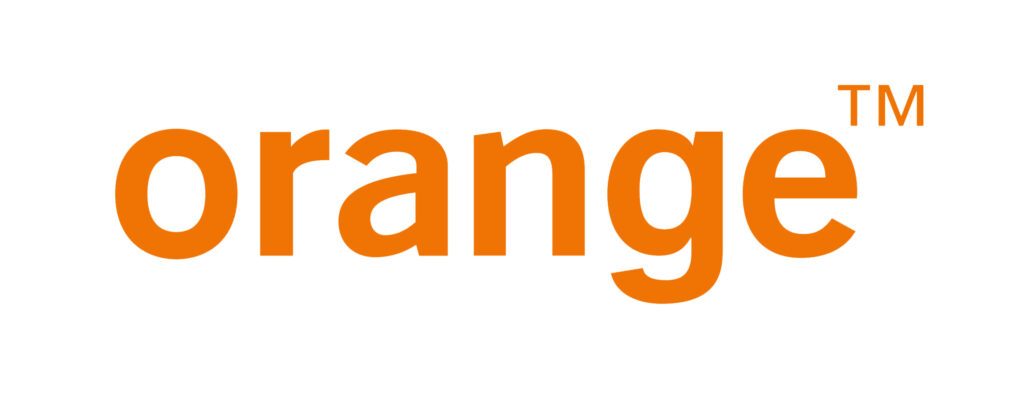
Features that Made Orange Shine
Orange’s success can be attributed to its impressive array of features. Here are some of the standout ones:
1. Versatile Data Visualization
Orange allows you to visualize your data in a wide variety of ways, from scatter plots to interactive data tables. It’s a data storyteller’s dream. In the realm of data visualization, Orange stands as a true artist’s palette. Its wide array of visualization options isn’t just about presenting data; it’s about crafting narratives. Scatter plots reveal hidden relationships, while interactive data tables provide in-depth exploration. Orange empowers you to unleash your creativity in data storytelling.
2. Robust Machine Learning
With a host of machine learning algorithms at your fingertips, Orange makes it easy to build and evaluate predictive models. Data science thrives on the ability to predict outcomes, and Orange’s treasure trove of machine learning algorithms makes this endeavor accessible to all. Whether you’re predicting customer behavior, diagnosing diseases, or optimizing supply chains, Orange equips you with the tools needed to unlock insights and make informed decisions.
3. Visual Programming
The visual programming interface of Orange makes it accessible even to those with minimal coding experience. You can create complex workflows without writing a single line of code. Coding can be a daunting barrier for many aspiring data scientists. Orange breaks down that barrier with its intuitive visual programming interface. It’s like building a complex puzzle; you connect the pieces, and Orange handles the intricate coding in the background. This empowers a broader range of professionals to harness the power of data science.
4. Extensive Add-ons
Orange’s add-on ecosystem is rich, providing additional functionality for various data analysis tasks. Data analysis is a multifaceted field, and Orange acknowledges this diversity through its extensive library of add-ons. Whether you need to analyze text data, perform network analysis, or dive into deep learning, there’s an add-on tailored to your specific analytical needs.
Limitations of Orange
As powerful as Orange is, it’s not without its limitations. Understanding these limitations can help you make an informed decision when searching for an Orange alternative:
1. Learning Curve
While Orange is user-friendly, mastering all its features can take time, especially for beginners. Orange is akin to an intricate musical instrument. While it’s designed to be user-friendly, becoming a virtuoso takes time and practice. For those new to data science, the learning curve may appear steep, but the journey is rewarding, as it opens doors to the world of data-driven insights.
2. Scalability
Orange may struggle with extremely large datasets, making it less suitable for big data projects. In the era of big data, scalability is paramount. Orange excels in handling moderate-sized datasets, but when confronted with massive volumes of information, its performance may begin to wane. This limitation prompts users dealing with substantial data to explore Orange alternatives designed to scale gracefully.
3. Limited Deep Learning Support
If you’re heavily into deep learning, Orange’s capabilities in this area might not be sufficient. Deep learning is the heart of cutting-edge artificial intelligence, and while Orange offers a plethora of machine learning tools, its deep learning support is comparatively limited. For those diving deep into neural networks, convolutional layers, and recurrent networks, specialized deep learning platforms may be more suitable.
4. Non-Commercial Use
Some users may be deterred by Orange’s licensing for commercial purposes. While Orange is a freely available tool for non-commercial use, its licensing terms for commercial purposes may pose challenges for businesses. For those operating in commercial domains, exploring Orange alternatives with more permissive licensing may be a sensible choice.
Why Seek an Orange Alternative?
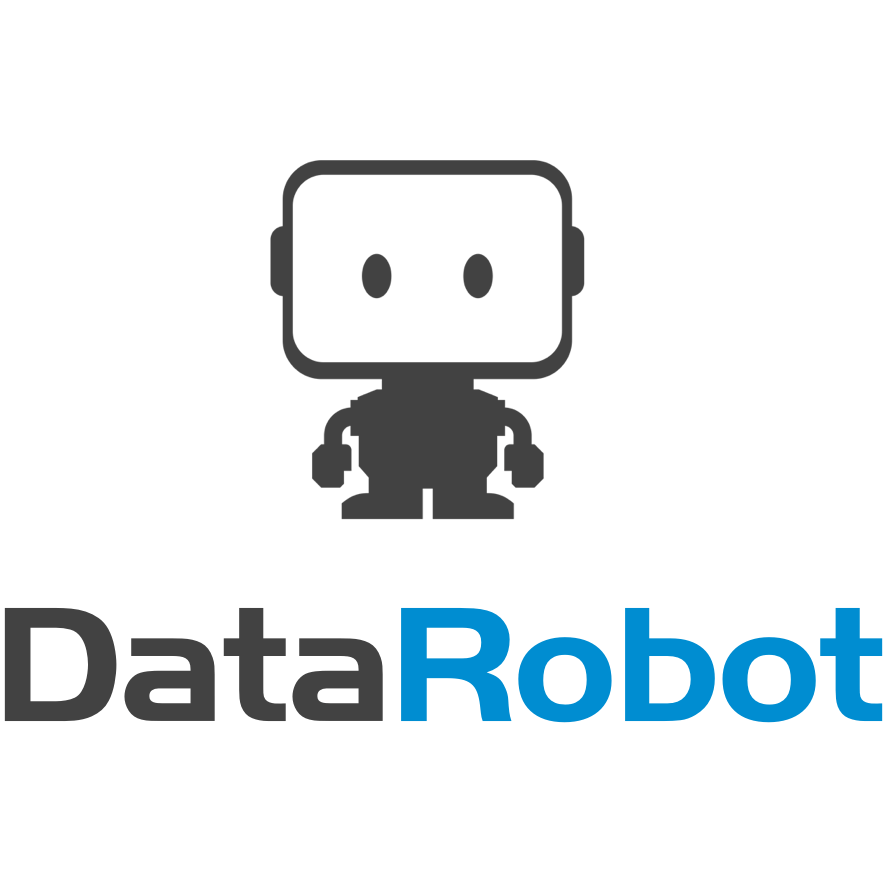
Despite Orange’s strengths, there are various reasons why one might look for an Orange alternative:
1. Diverse Needs
Different projects may require specialized tools that Orange might not offer. In the realm of data analysis, no two projects are identical. Some may demand specialized functionalities not readily available in Orange. Whether you’re conducting sentiment analysis on social media data or simulating financial scenarios, seeking an Orange alternative tailored to your specific needs can be a game-changer.
2. Scalability
For handling large-scale data analysis, alternatives with better scalability may be preferred. Data’s growth knows no bounds, and as your datasets expand, so must your analysis tools. Choosing an Orange alternative that excels in scalability ensures that you can tackle projects of any magnitude with confidence.
3. Cost Concerns
Budget constraints may drive users to seek free or more cost-effective options. In the world of data analysis, budgets can be tight. If the cost of using Orange, especially when combined with its add-ons, exceeds your financial limits, exploring Orange alternatives that align better with your budget is a prudent decision.
Commonly Used Orange Alternatives for Data Analysis
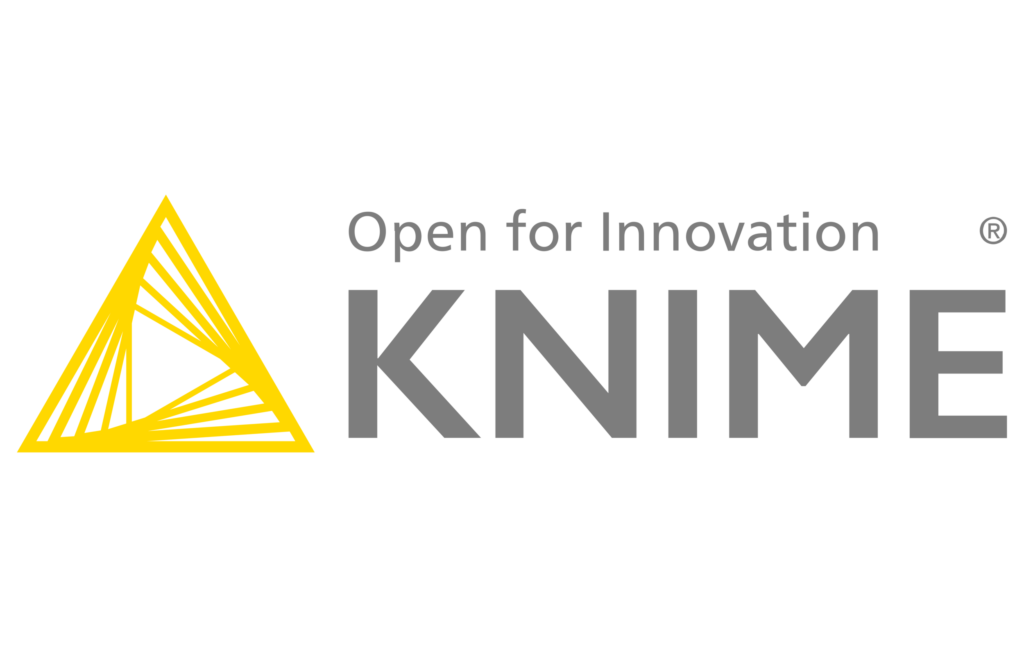
Now, let’s delve deeper into some commonly used Orange alternatives, each tailored to specific data analysis needs:
1. KNIME
KNIME is a powerful open-source data analytics platform that offers a wide range of data manipulation, analysis, and visualization tools. KNIME’s feature set is extensive, making it a formidable competitor in the data analysis arena. Its visual data integration and transformation capabilities streamline data preprocessing.
With an extensive library of data mining and machine learning algorithms, it provides the building blocks for predictive modeling, classification, and clustering tasks. KNIME’s strength lies in its flexibility and compatibility, thanks to its integration with various data sources and databases. Whether you’re working with structured or unstructured data, KNIME’s adaptability ensures that you can seamlessly access and analyze your data.
2. RapidMiner
RapidMiner is a user-friendly, open-source data science platform that simplifies data analysis and predictive modeling. RapidMiner is designed with ease of use in mind, making it an ideal choice for both beginners and experienced data scientists. Its intuitive drag-and-drop interface empowers users to construct analysis pipelines effortlessly.
Supporting a wide array of data formats, RapidMiner ensures that you can work with data from various sources. What sets RapidMiner apart is its extensive machine-learning and text-mining capabilities. From decision trees and neural networks to sentiment analysis and natural language processing, RapidMiner equips you with the tools to tackle diverse analytical challenges.
3. Weka
Weka is a collection of machine-learning algorithms for data mining tasks. It provides a graphical user interface for creating and evaluating machine learning models. Weka, while known for its simplicity, offers a rich set of machine-learning tools. Its collection of over 100 machine-learning algorithms covers a wide spectrum of tasks.
Data preprocessing and visualization tools assist in data understanding and cleaning, paving the way for more accurate models. Weka’s integration with the Waikato Environment for Knowledge Analysis (Weka) ecosystem expands its capabilities further. It’s a choice that combines user-friendliness with substantial analytical power.
4. DataRobot
DataRobot is a commercial automated machine-learning platform designed to streamline the machine-learning process. DataRobot takes automation to the forefront of machine learning. Its automated model selection and optimization enable data scientists to build and fine-tune models rapidly.
Beyond traditional machine learning, DataRobot’s support for time-series analysis and natural language processing empowers users to explore a wider range of data types. The platform’s ease of use extends to model deployment, making it a comprehensive solution for organizations seeking efficiency and automation in their data analysis pipeline.
5. Python Libraries (Scikit-learn, Pandas, Matplotlib)
Python offers a vast ecosystem of libraries for data analysis, including Scikit-learn for machine learning, Pandas for data manipulation, and Matplotlib for data visualization. Python’s strength lies in its versatility and extensive community support. Scikit-learn, one of Python’s most renowned libraries, boasts a wide range of machine-learning algorithms for classification, regression, clustering, and more.
Pandas, another Python gem, excels in data manipulation, allowing you to clean, transform, and preprocess data efficiently. Matplotlib, a go-to library for data visualization, provides the means to create stunning graphs, charts, and plots to convey your insights effectively. The integration of these libraries with other data science tools gives you a flexible and customizable toolkit for data analysis.
6. Tableau
Tableau is a commercial data visualization tool known for creating interactive and shareable dashboards. Tableau’s strength lies in its ability to turn data into visual stories. Its user-friendly drag-and-drop interface empowers individuals across organizations to create interactive dashboards and reports without requiring coding expertise.
The integration of various data sources allows you to access and analyze data from multiple platforms, databases, and cloud services. Real-time data visualization capabilities provide up-to-the-minute insights, making Tableau a valuable asset for organizations seeking to harness the power of data-driven decision-making.
7. Power BI
Microsoft Power BI is a commercial business intelligence tool for data visualization and reporting. Power BI’s affiliation with the Microsoft ecosystem makes it a compelling choice for organizations already utilizing Microsoft technologies. Its integration with Excel, Azure, and other Microsoft services streamlines data access and analysis.
Interactive dashboards and reports facilitate data exploration and sharing. Furthermore, Power BI’s AI-powered insights and recommendations elevate data analysis by automatically identifying trends, anomalies, and correlations, allowing users to derive deeper insights from their data.
8. QlikView/Qlik Sense
QlikView and Qlik Sense are commercial data visualization and business intelligence tools with a focus on associative data modeling. QlikView and Qlik Sense distinguish themselves with their associative data model, which enables dynamic data exploration. These tools allow users to interact with data intuitively, drilling down into details and instantly spotting patterns and relationships.
The drag-and-drop interface simplifies the creation of interactive dashboards and reports, making data analysis more accessible to a wider audience. With multi-cloud deployment options, organizations can choose the deployment model that best suits their needs, ensuring flexibility and scalability.
9. Alteryx
Alteryx is a commercial platform for data blending, preparation, and advanced analytics. Alteryx specializes in data preparation and blending. Its data blending and cleaning capabilities enable users to combine data from multiple sources efficiently.
Geospatial analytics add an extra dimension to data analysis, making it ideal for location-based insights. Furthermore, Alteryx offers predictive and prescriptive analytics tools, allowing organizations to forecast trends, optimize processes, and make data-driven decisions with confidence.
10. Orange3
Orange3, an updated version of Orange, offers an open-source, visual programming interface for data analysis and machine learning. Orange3 builds on the strengths of its predecessor. Its visual workflow design remains its hallmark, making it accessible to individuals with varying levels of coding expertise.
The extensive library of data analysis and machine learning components ensures that you have a wide array of tools at your disposal. Furthermore, Orange3’s integration with Python extends its capabilities, providing the flexibility to incorporate Python’s vast ecosystem of libraries and packages into your data analysis projects.
Each of these Orange alternatives brings its unique strengths to the table, catering to a wide range of data analysis needs. Whether you’re looking for a robust machine learning platform like KNIME or a user-friendly interface like RapidMiner, the options are plentiful.
Factors to Consider While Choosing the Perfect Orange Alternative
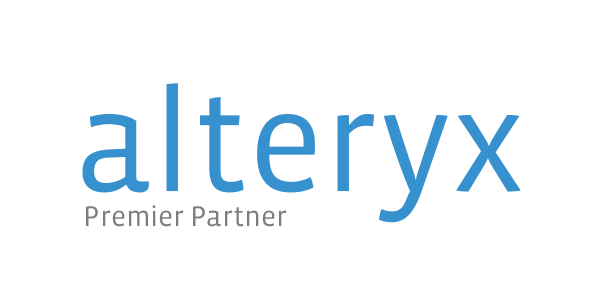
Choosing the right Orange alternative depends on your specific needs and preferences. Here are some factors to consider:
1. Project Requirements
Assess your project’s specific data analysis and visualization requirements. Every project has its nuances, and understanding the precise needs of your analysis is the first step in selecting the perfect alternative. Consider the types of data you’re working with, the complexity of your analysis, and your end goals.
2. Learning Curve
Consider your team’s familiarity with the tool and the time required for onboarding. Time is money, and a steep learning curve can slow down your projects. Evaluate the skill levels of your team members and weigh it against the tool’s ease of use. A quicker onboarding process can lead to faster results.
3. Scalability
Evaluate whether the alternative can handle the size of your datasets. Data is growing exponentially, and your tool should be able to keep up. If you’re dealing with large datasets or anticipate growth in your data volume, scalability becomes a critical consideration.
4. Budget
Factor in the cost of the tool and its licensing terms. Budget constraints are a reality in most organizations. Carefully analyze the cost structure of your chosen alternative, including any licensing fees, maintenance costs, and potential scalability-related expenses.
5. Ecosystem
Check if the alternative integrates well with your existing tools and workflows. Data analysis rarely happens in isolation. Ensure that your chosen alternative can seamlessly integrate with your existing software stack. Compatibility with data storage solutions, databases, and other tools is crucial for a smooth workflow.
Conclusion
In the world of data analysis, having the right tools at your disposal can make all the difference. While Orange has undoubtedly been a valuable asset to many, exploring alternatives can open up new possibilities and cater to diverse needs. Whether you opt for KNIME’s versatility, RapidMiner’s user-friendliness, or the extensive Python ecosystem, there’s an Orange alternative waiting to help you achieve your data analysis goals.
As you embark on your journey to find the perfect Orange alternative, keep in mind that the best choice depends on your unique circumstances. Consider your project’s requirements, your team’s skills, and your budget. With the right alternative in hand, you’ll be well-equipped to tackle the data analysis challenges that lie ahead.